Leveraging Synthetic Data
Synthetic data is data that's generated from computer simulations or algorithms to create AI (artificial intelligence) models. One of the biggest benefits to using synthetic data is the time and the cost it takes to generate usable data.
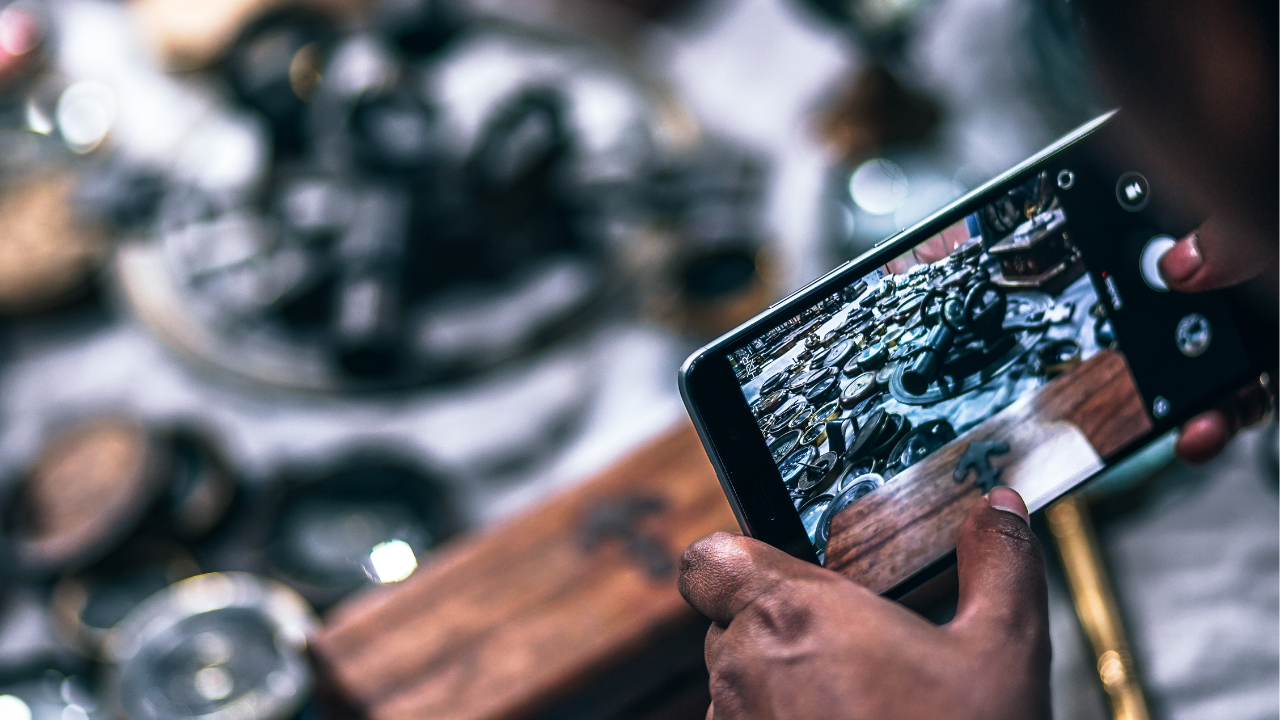
As we incorporate more artificial intelligence into health IT, the topic of synthetic data keeps getting more attention. In this video I will touch on what synthetic data is and why we should consider using more of it in healthcare.
Video:
Video Transcript:
Hi there, I'm Drae. The host and founder of the Draegan Network. On this week's YouTube video I would like to chat about synthetic data. what exactly it is and how it factors into our health IT space. Before I get started, if you haven't subscribed to this channel yet, don't forget to do that before you finish watching the video. And as always if you like the content don't forget to hit the like button.
[00:23] Synthetic data is data that's generated from computer simulations or algorithms to create AI (artificial intelligence) models. Essentially it is information that is created within the digital space instead of within the real world. When I first heard the term I was a little bit confused … but it is exactly the same as synthetic materials or synthetic medications. It is something that instead of being created in the natural space, it is developed inside of a lab. In this case it just happens to be a computer lab and it is computer simulations and algorithms that are generating that data. The exact same concept is there so the data that's generated is going to reflect the real world data from a mathematical and a statistical perspective…because it's generated from simple rules, statistical modeling, simulation, and other techniques.
[01:22] One of the things that's important about creating synthetic data is the larger the data set is that you have to work with (the larger the data set inside the neural network) the easier it is to train the algorithms and the simulations to actually generate the synthetic data. So, you need a huge amount of data to start with.
[01:43] Why would we use synthetic data? Where would it actually factor in? In order to build high-value, high-quality artificial intelligence models we know that there is some augmentation that will need to occur. We're not going to be in a situation where that AI model can have every single piece of information that it needs presented to it in a real world form. If you take a look at pictures for example (digital photos). We often will take a photo and we're going to go to a program to maybe touch it up a little bit. Lighten the background. Do things like that. That is all additional augmented data that's being incorporated into the image. In a very appropriate, defined, and contextual way to augment and present a more realistic photo. That's the same concept that we're going to think of in the health IT space.
[02:33] One of the biggest benefits to using synthetic data is the time and the cost it takes to generate the data. If you think of the amount of data that's required to train neural networks and artificial intelligence systems on a global scale. To accurately predict, diagnose, and assist in a healthcare space. That collection and that data analysis, if it's done by humans, is astronomically expensive and extremely time consuming. Just think of all those times you've had to collate information onto a single spreadsheet and how long that actually took. With synthetic data we can generate volumes of data that are again mathematically sound and statistically relevant, and simulate those real world data points much faster and much cheaper.
[03:23] Synthetic data also allows you to mitigate privacy issues. There are a number of industries, of course health being one of those, where privacy is first and foremost on our minds in all situations. If we're looking at information about an individual's health we want to make sure that individual’s privacy is protected. With synthetic data a lot of those concerns can be alleviated because the information isn't actually tied to an actual real-world person. It is a model or a simulation of an individual but it doesn't correlate back to a human.
[04:00] In theory, synthetic data can also reduce bias in your AI algorithms and in your data samples. Now that of course is going to depend on how it was actually set up and how that synthetic data was generated; but, there is an opportunity for the individuals that are programming to be much more mindful of any biases that may be introduced and to factor those into all of the programming. As opposed to in the real world where our personal biases, regardless of how hard we try to keep them out… are going to in some instances influence our data collection. They're going to influence our thought patterns and things like that. So synthetic data, theoretically if it's done right and if it's programmed correctly, can alleviate some of those concerns.
[04:45] [With synthetic data] we're going to go after the privacy concerns. It can go after any volume concerns, cost concerns, and also the bias. Synthetic data, right now as you can imagine, is a growing space. The data sets that are available are not 100% accurate but there's absolutely a lot of work that's being done in the space. Individuals are consistently trying to make that data better. In the healthcare industry there are some organizations that are currently using fully synthetic patient data, and fully synthetic claims data, in some of their AI development, in some of their projects, and in various other ways. It again is not linked back to an individual patient; so, if there's a breach of synthetic data there is absolutely no way that it can be tied back to an actual human because that's not who it's based on. When synthetic data is used it does need to be consistently developed, calibrated, and validated against real-world data. You definitely don't want to be in a situation where you've generated synthetic data that doesn't reflect real world data and be using that for anything in the healthcare space. The evaluation and validation of those data sets, and of the creation of the synthetic data, is incredibly important here.
[05:56] You might be wondering, if we don't have a lot of AI algorithms or deep neural networks in place, why would we need to use synthetic data? Or why would we consider using synthetic data? One of the best applications that people can start with is utilizing synthetic data in the testing process. If you think of your test environment for your EHR or for your ancillary applications. There is a consistent struggle to populate the test environment with enough information so that you can actually validate and functionally test the information that you need before you roll it out to production. A lot of times we're looking at things like scramble-scripts or strip-scripts to try to remove all the patient data and the patient information. But when we do that we're not necessarily getting the exact real world situation that we might want. A scramble script for example, can scramble things too much. Where some of our clinical decision support isn't actually going to make much sense or we're not really going to be able to try it. With synthetic data you could model your test environment after a real-world situation and fully populate it with details that are statistically and mathematically relevant. To reflect real world space to do your testing.
[07:13] I think that's super exciting because I've had to spend many, many hours over the course of my career populating test environments and populating training environments. If we could fill that up with synthetic data, that would be fabulous! (We wouldn't have to worry about copying the patient information from prod to test again, so the privacy and security people would be pretty excited as well.) Synthetic data is definitely a world that's growing in every single industry. Health IT is no exception. We're certainly going to see more applications of this and as more data sets are generated, and as more data sets are validated, the way that they can be leveraged is actually pretty exciting.
[07:49] I hope that today's video was helpful and gave you an idea of what synthetic data is and how synthetic data may be able to be utilized in the healthcare IT space. Again if you haven't subscribed to the channel, don't forget to do that before you go. I will see you again next time.